Physics Informed Neural Networks for Vortex-Induced Vibration: Experimental Insights with Tunable Stiffness
Keywords:
vortex-induced vibration, physics-informed neural networks, fluid-structure interaction, modal participationAbstract
Vortex-induced vibration (VIV) of cylindrical structures is a complex fluid-structure interaction phenomenon encountered in many engineering applications. Computational modeling of VIV remains challenging due to the need to accurately capture unsteady wake dynamics and fluid-structure coupling effects. In this work, we develop a physics-informed neural network (PINN) framework for data-efficient and accurate VIV modeling and prediction. The key novelty lies in incorporating fluid dynamic principles directly into the PINN architecture through automatic differentiation. This obviates the need for extensive labeled data encompassing the parameter space for training. Furthermore, experimental insights into VIV modal interaction are incorporated through tunable structural stiffness in a segmented cylinder experimental setup. Nonlinear modal participation factors are proposed to quantify mode-switching behavior. The results demonstrate that the PINN model can accurately capture lock-in occurrence, predict amplitude response, and model higher harmonic responses using limited experimental data. The PINN model also provides smooth approximations of displacements and fluid forces, enabling the extraction of fluid damping coefficients. This work provides a foundation for data-efficient, physics-embedded neural network modeling of complex dynamical systems.
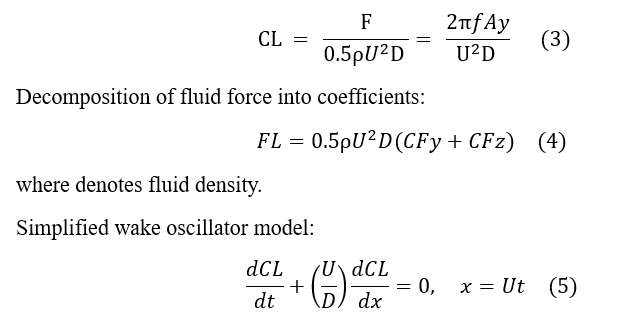