Deep Learning and Computer Vision-Based Retail Analytics for Customer Interaction and Response Monitoring
Keywords:
Computer Vision, Customer Interaction, Deep Learning, Foot Traffic, Heatmaps, Retail Analytics, Response MonitoringAbstract
Traditional methods of retail analytics have limitations in capturing detailed customer interactions and responses within the store environment. This gap in data collection and analysis can lead to inefficiencies in store layout design, product placement, and promotional activities. The current study investigates the potential of deep learning and computer vision technologies to enhance retail analytics. This research presents an in-depth exploration of the application of deep learning-based computer vision technologies in enhancing retail analytics. The study is structured around four principal aspects: Analysis of Dwell Times, Assessment of Foot Traffic, Generation of Customer Movement Heatmaps, and Evaluation of Customer Responses to In-Store Promotions. Each aspect is thoroughly examined through the lenses of purpose, practical application, advantages, system components, and their interconnectivity, offering a detailed perspective on the operational dynamics and benefits of these technologies. The research discusses the importance of measuring customer engagement in specific store areas. The deployment of a sophisticated system comprising strategically located cameras, sensors, data processing units, and analysis software facilitates the acquisition of valuable data. This system is used in enhancing store layout, product positioning, and resource allocation by leveraging accurate data on customer interest in different sections. The aspect of Foot Traffic Analysis involves the use of cameras and sensors at store entrances and throughout the premises. This setup enables the quantification of customer entries, exits, and in-store movements, providing essential awareness into peak-activity periods and movement patterns. The system's capacity for data aggregation and traffic pattern analysis can optimize staff deployment and store layout, thereby augmenting the overall effectiveness of store displays. The creation of heatmaps of customer movement uses data from overhead cameras to visualize high-traffic areas within the store. This approach aids in pinpointing areas of high customer engagement, facilitating strategic product placement and the identification of areas requiring improvement. Evaluating customer reactions to in-store promotions involves computer vision techniques for analyzing facial expressions, body language, and gaze direction, providing evaluation of the efficacy of promotional strategies. This system, encompassing facial recognition cameras, body language analysis tools, and sentiment analysis software, enables retailers to tailor their marketing approaches based on real-time customer feedback. The research also addresses the challenges accompanying the adoption of these technologies. Concerns about customer privacy and the intrusive nature of continuous monitoring are significant. Moreover, the collection and processing of personal data, particularly in the context of facial recognition, necessitate careful consideration of consent and privacy issues. Retailers must also adhere to data protection laws like GDPR, to ensure lawful and ethical use of these technologies for retail analytics.
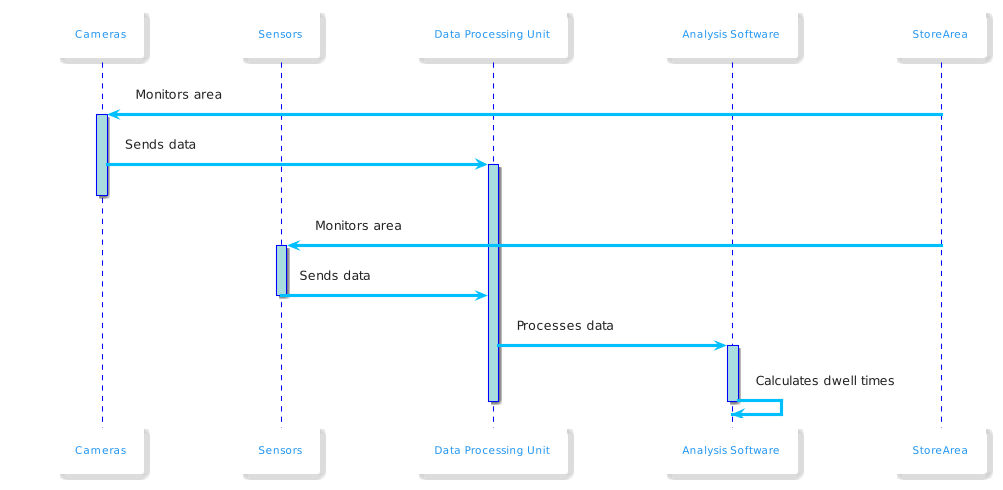